Maximizing Efficiency: The Pivotal Role of AI in Modern Healthcare
AI has the potential to improve how quickly we're able to access important information and deliver care.
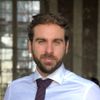
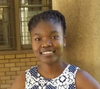
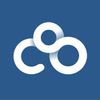
Published December 24, 2023.
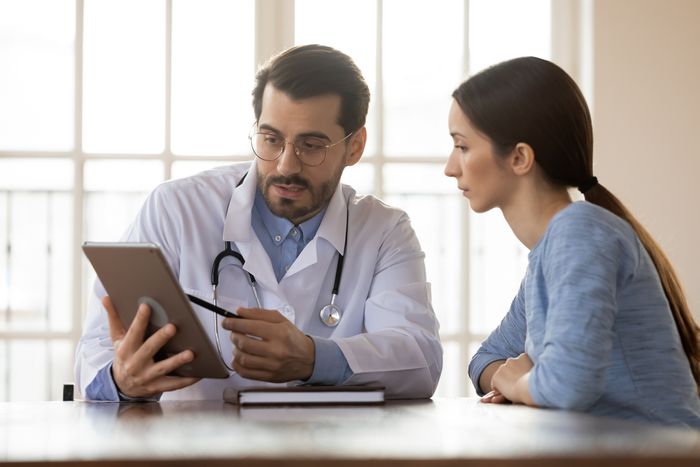
Artificial Intelligence (AI) has become a transformative force in many sectors, and healthcare is no exception. As innovation continues to drive healthcare forward, investment in AI within the field is growing—estimated to reach $187.95 billion by 2030.
Amid a sea of clinician documentation, generative AI tools in particular can help healthcare providers at every step, from quickly accessing trusted knowledge to diagnosing and treating illnesses, and ultimately saving lives. Here's how AI can play a pivotal role in modern healthcare.
Ido Zamberg M.D. is a board-certified physician (General Internal Medicine and Anesthesia), currently a Fellow at the Division of Experimental Medicine, McGill University Health Center, Montreal, Canada.
Enhancing Operational Efficiency
Healthcare professionals handle vast amounts of diverse information daily. They need easy access to necessary knowledge for quick decision-making. Generative AI can streamline this process by scanning and analyzing notes, statistical information, and documentation, bringing essential clinical material to the forefront when and where it's needed.
Take the Enhanced Recovery After Surgery (ERAS) protocol for example. These protocols aim to decrease the length of stay and enhance the overall patient experience through a multimodal and multi-disciplinary strategy. It includes specific guidelines for the preoperative, intraoperative, and postoperative stages of care.
Traditionally, ERAS protocols relied on statistical models to analyze patient data. However, a shift towards artificial intelligence models has demonstrated improvements in operational efficiency. For instance, one study showed that AI models resulted in better management of hospital length of stay compared to statistical models.
By integrating AI into the ERAS protocol, healthcare providers can improve operational efficiency, reduce costs, and enhance patient care.
» Looking to curb healthcare spend? Check out how to reduce healthcare costs
Predictive Modeling and Diagnosis
Machine learning, a subset of AI, is increasingly used in healthcare for predictive modeling. Using large datasets, supervised machine learning is applied for various healthcare problems such as disease diagnosis and treatment selection. Additionally, it can predict disease outcomes and patient trajectories.
A notable application of machine learning in healthcare is predicting sepsis, a life-threatening condition that arises when the body’s response to infection injures its tissues and organs.
Machine learning algorithms can analyze many clinical parameters, including vital signs and lab values, to predict the onset of sepsis up to 48 hours before it occurs. This early prediction allows for timely intervention, potentially saving lives and improving patient care.
The ability to predict sepsis 24 hours in advance is a significant advancement in healthcare, as it provides healthcare professionals with a crucial window for intervention, potentially improving patient outcomes and reducing healthcare costs.
» See how C8 Health can make life easier for your medical team.
Imaging Analysis for Treatment Planning
With hospitals today performing 3.6 billion imaging procedures annually, generating a massive amount of data, AI is revolutionizing imaging analysis in healthcare. It analyzes imaging data from CT scans and MRIs to assist healthcare professionals in treatment planning.
For instance, in stroke patients, AI is used to analyze CT scans to determine the size and location of the lesion.
This information is crucial in deciding the appropriate treatment strategy. By automating this process, AI enhances efficiency and reduces the likelihood of human error, leading to more accurate diagnoses and treatment plans.
The use of AI in imaging analysis also significantly reduces the time taken for analysis, thereby improving the efficiency of the healthcare system and possibly reducing staff burnout.
» Explore other ways to improve staff wellness
Information Retrieval
Having centralized knowledge management systems can greatly improve information retrieval in healthcare, allowing professionals to quickly and efficiently find answers to clinical questions.
With its natural language search capabilities, AI can expedite this process by quickly retrieving relevant and vetted information from a knowledge base. This improves the efficiency of clinical operations and ensures that healthcare professionals have access to the most accurate and up-to-date information.
The C8 Health platform draws on the power of AI for quick and accurate information retrieval from a vast knowledge base, so you're able to get concrete answers to your questions quickly without sifting through reams of research.
AI Considerations in Modern Healthcare
The worlds of tech and healthcare can complement each other well, especially when it comes to distilling the breadth of information that clinicians need to make decisions—and making that information more accessible than ever.
As we increase our dependency on these technologies, it's important to ensure that healthcare professionals remain well-trained and informed for effective risk management.
As we continue to explore and implement AI in healthcare, we can look forward to a future where healthcare is more efficient, predictive, and personalized. The potential of AI in healthcare is immense, and the right approach can lead to significant improvements in patient care and operational efficiency.
» Here's how C8 Health helps streamline your organization's operations.