Innovation in Healthcare: 5 Examples of AI-Driven Breakthroughs
AI is no longer science fiction; it's at the forefront of innovation in healthcare. Explore five incredible examples of how this technology is transforming medicine.
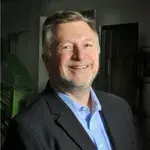
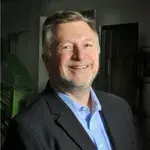
Mical DeBrow (Ph.D., RN) is a highly accomplished healthcare professional with a wealth of hands-on experience spanning various sectors of the industry. With a deep understanding of operations, policy implementation, and education in clinical settings, Mical brings a unique perspective to...
See Full BioLearn about our
Editorial PolicyPublished August 5, 2024.
The healthcare industry is in trouble. Costs are skyrocketing, there are more old people than ever, and diseases are getting trickier to treat. Patients are paying more for care, and doctors are working harder with fewer resources at their disposal.
We need a big change if we're to deliver better, faster, and more personal care. AI may just be the solution we've been waiting for. That's why we've prepared some examples of how it's driving innovation in healthcare.
» Lead healthcare innovation by using a knowledge management system
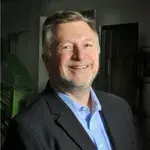
1. Accurate Diagnosis for Patient Safety
Even with all our modern medicine, early disease detection is still a considerable challenge. Every illness is different and affects each person uniquely. It was almost impossible to create a single tool for it-until now.
Machine learning-a branch of AI-brought innovation in this field of healthcare. While it's still new, it may help in several ways:
- A South Korean study showed that AI was more sensitive in diagnosing breast cancer than radiologists. [1]
- Research says that deep learning algorithms are also more sensitive when finding pneumonia in chest X-rays. [2]
- Experts are actively testing AI in analyzing X-rays, CT scans, and MRIs. It may help us quickly find abnormalities, fractures, and tumors. [3,4]
- In clinical microbiology, deep learning algorithms can classify strains. [5,6] Some of them could also find malaria-infected blood cells without staining.
- AI innovation in genomic medicine shows promise for tracking new threats like COVID-19. [7] Algorithms can detect complex patterns of genes and predict simple traits or drug responses.
AI can help speed up decision-making pipelines. Watch our latest webinar to learn more.
» Check out different types of health information technology
2. Tailored Treatment for Better Health Outcomes
Experts are finding new ways to treat people by giving them the correct drugs for their bodies. This is called personalized medicine.
AI is driving healthcare innovation in this field. It analyzes complex datasets and predicts outcomes to optimize treatment. [8] Studies have shown that it can predict chemotherapy and antidepressant responses using genetic and EHR data. [9]
While progress is promising, more research is needed to refine AI algorithms' reliability in real-world settings.
» Use data-driven insights for tailored patient care
3. Knowledge Management for Operational Efficiency
AI-powered knowledge management systems drive healthcare innovation in several ways:
- Data Analysis: AI can process vast amounts of medical data, finding patterns that human analysts might miss. Clinicians can gain new insights, make decisions easier, and develop evidence-based practices.
- Knowledge Discovery: AI can help unearth valuable information hidden within medical records, research papers, and clinical notes.
- Personalized Learning: Platforms can tailor learning modules to healthcare professionals based on their roles and expertise.
- Efficient Knowledge Sharing: Some AI tools can simplify knowledge sharing by automating content grouping, tagging, and recommendation.
- Natural Language Processing (NLP): Knowledge management systems like C8 Health use NLP to understand and process human language, which helps your care teams quickly find information by asking questions.
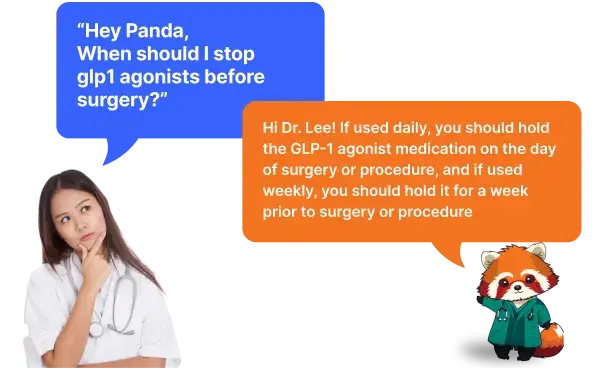
4. Custom Medication Therapy for Reduced Errors
AI predictive analytics can find at-risk populations and guide targeted interventions by [10]:
- Looking at data to predict chronic disease development or hospital readmissions.
- Tailoring medication dosages.
- Predicting side effects, reducing risks, and improving patient outcomes. [11]
A study created an AI-based model to predict prothrombin time international normalized ratio (PT/INR) and optimize warfarin dosing. [12] The algorithm outperformed expert physicians.
AI is also driving innovation in the healthcare industry when it comes to therapeutic drug monitoring. [13] Here's how:
- Machine learning algorithms predict drug-drug interactions by analyzing large patient datasets.
- Predictive analytics find people at risk of adverse drug reactions. Healthcare providers can use them to take preventative measures.
» Increase staff's best practice adherence
5. Predictive Analytics for Global Health
AI algorithms can also uncover hidden health trends simply by looking at what people are saying online. It turns out that the casual conversations we have on social media can offer valuable insights into the health of a nation.
Sehaa-a big data analytics tool used in Saudi Arabia-can detect diseases through information found on Twitter. [14] It identified hypertension, cancer, diabetes, and dermal and heart disease as the top five most prominent in the country's population.
» Check out the top CRM functionalities for healthcare
Challenges to AI and Technology Adoption
AI might drive innovation in healthcare, but it also faces some challenges that may hinder adoption, such as:
Staff Resistance
Healthcare providers are cautious about new technology. [15] They may be worried about the reliability of AI algorithms, job displacement, and the effort required to adapt these tools to their workflow.
The way AI systems interact with doctors also influences adoption. Too much information or frequent alerts can overwhelm clinicians, so you should find one that's made with the user in mind.
To build trust, you would need to invest in continuous training and education. You should also show your staff the tangible benefits of care innovations driven by AI technology.
High Initial Costs
Research shows that AI could reduce the costs of US healthcare by 5-10%-that's roughly $200-360 billion yearly. [16] These savings predictions are based on the technology we have and can put to use within the next five years.
As more facilities adopt these technologies, competition will grow. Prices will decrease, making AI accessible to everyone.
So, what does prevent us from using it right now? The initial cost of implementing this solution creates a barrier for healthcare organizations with limited resources. Hardware, software, training, and ongoing maintenance would all need to be paid for.
» Reduce healthcare costs through knowledge management systems
Privacy and Security Vulnerabilities
Protecting the business side of AI and data-driven health technologies is becoming crucial. [17] Traditionally, only doctors could evaluate a person's health by tracking blood pressure and heart rate. Now, different medical devices can do it.
But what does that mean for patient privacy? Laws like HIPAA in the US and GDPR in Europe help protect their data. While HIPAA focuses on health information, GDPR offers broader data protection across Europe.
The UN General Assembly has also adopted an AI resolution. [18] Over 120 nations backed a plan for safe, secure, and trustworthy AI development.
Still, these laws don't stop third parties from exploiting weaknesses in your IT systems. You may be facing threats from cyberattacks if you decide to host sensitive patient data or hospital guidelines. In 2023, OCR reported a 239% increase in hacking-related data breaches. [19]
To reduce these risks, you must invest in advanced security technologies, implement access controls, and do regular security audits. Additionally, training your employees on cybersecurity best practices can prevent human error from becoming a vulnerability.
» Discover the benefits of cloud-based solutions for your organization
Future of Patient Care in a Digital World
AI is ushering in a new era of medical advancement. The five examples explored in this article are just a glimpse of how it encourages innovation in the healthcare industry.
As algorithms continue to evolve, their applications will expand. They will lead to more accurate diagnoses, personalized treatments, and improved patient outcomes. But, it's crucial to address data privacy and ethical concerns to ensure we use these tools responsibly.
Despite the challenges, the future of healthcare is undeniably intertwined with AI, and the journey ahead promises to be both exciting and challenging.
» See how C8 Health can make life easier for your medical team.
References:
- "Changes in cancer detection and false-positive recall in mammography using Artificial Intelligence: A retrospective, multireader study," The LANCET Digital Health, Available: https://www.thelancet.com/journals/landig/article/PIIS2589-7500(20)30003-0/fulltext
- J. Becker et al., "Artificial Intelligence-Based detection of pneumonia in chest radiographs," Diagnostics, vol. 12, no. 6, p. 1465, Jun. 2022, doi: 10.3390/diagnostics12061465. Available: https://www.mdpi.com/2075-4418/12/6/1465
- S. J. Adams, R. D. E. Henderson, X. Yi, and P. Babyn, "Artificial intelligence solutions for analysis of X-ray images," Canadian Association of Radiologists Journal, vol. 72, no. 1, pp. 60-72, Aug. 2020, doi: 10.1177/0846537120941671. Available: https://pubmed.ncbi.nlm.nih.gov/32757950/
- R. Paudyal et al., "Artificial intelligence in CT and MR imaging for oncological applications," Cancers, vol. 15, no. 9, p. 2573, Apr. 2023, doi: 10.3390/cancers15092573. Available: https://www.ncbi.nlm.nih.gov/pmc/articles/PMC10177423/
- K. P. Smith, A. D. Kang, and J. E. Kirby, "Automated interpretation of blood culture gram stains by use of a deep convolutional neural network," Journal of Clinical Microbiology, vol. 56, no. 3, Mar. 2018, doi: 10.1128/jcm.01521-17. Available: https://doi.org/10.1128/jcm.01521-17
- "Machine learning in the Clinical Microbiology Laboratory: Has the time come for routine practice?," Clinical Microbiology and Infection, Available: https://www.clinicalmicrobiologyandinfection.com/article/S1198-743X(20)30085-9/fulltext
- S. A. Bagabir, N. K. Ibrahim, H. A. Bagabir, and R. H. Ateeq, "Covid-19 and Artificial Intelligence: Genome sequencing, drug development and vaccine discovery," Journal of Infection and Public Health, vol. 15, no. 2, pp. 289-296, Feb. 2022, doi: 10.1016/j.jiph.2022.01.011. Available: https://www.sciencedirect.com/science/article/pii/S1876034122000144?via%3Dihub
- S. Quazi, "Artificial intelligence and machine learning in precision and genomic medicine," Medical Oncology, vol. 39, no. 8, Jun. 2022, doi: 10.1007/s12032-022-01711-1. Available: https://doi.org/10.1007/s12032-022-01711-1
- C. Huang et al., "Machine learning predicts individual cancer patient responses to therapeutic drugs with high accuracy," Scientific Reports, vol. 8, no. 1, Nov. 2018, doi: 10.1038/s41598-018-34753-5. Available: https://www.nature.com/articles/s41598-018-34753-5
- K. M. Nelson, E. T. Chang, D. M. Zulman, L. V. Rubenstein, F. D. Kirkland, and S. D. Fihn, "Using predictive analytics to guide patient care and research in a national health system," Journal of General Internal Medicine, vol. 34, no. 8, pp. 1379-1380, Apr. 2019, doi: 10.1007/s11606-019-04961-4. Available: https://doi.org/10.1007/s11606-019-04961-4
- G. L. Martin et al., "Validation of artificial intelligence to support the automatic coding of patient adverse drug reaction reports, using nationwide pharmacovigilance data," Drug Safety, vol. 45, no. 5, pp. 535-548, May 2022, doi: 10.1007/s40264-022-01153-8. Available: https://doi.org/10.1007/s40264-022-01153-8
- H. Lee, H. J. Kim, H. W. Chang, D. J. Kim, J. Mo, and J.-E. Kim, "Development of a system to support warfarin dose decisions using deep neural networks," Scientific Reports, vol. 11, no. 1, Jul. 2021, doi: 10.1038/s41598-021-94305-2. Available: https://www.nature.com/articles/s41598-021-94305-2
- F. Sjövall, C. Lanckohr, and H. Bracht, "What's new in therapeutic drug monitoring of antimicrobials?," Intensive Care Medicine, vol. 49, no. 7, pp. 857-859, May 2023, doi: 10.1007/s00134-023-07060-5. Available: https://doi.org/10.1007/s00134-023-07060-5
- S. Alotaibi, R. Mehmood, I. Katib, O. Rana, and A. Albeshri, "SEHAA: a big data analytics tool for healthcare symptoms and diseases detection using Twitter, Apache Spark, and machine learning," Applied Sciences, vol. 10, no. 4, p. 1398, Feb. 2020, doi: 10.3390/app10041398. Available: https://www.mdpi.com/2076-3417/10/4/1398
- M. I. Ahmed, B. Spooner, J. Isherwood, M. Lane, E. Orrock, and A. Dennison, "A Systematic review of the barriers to the implementation of artificial intelligence in healthcare," Cureus, Oct. 2023, doi: 10.7759/cureus.46454. Available: https://www.ncbi.nlm.nih.gov/pmc/articles/PMC10623210/
- N. Sahni, G. Stein, R. Zemmel, and D. Cutler, "The potential impact of artificial intelligence on healthcare spending," Jan. 2023. doi: 10.3386/w30857. Available: https://www.nber.org/papers/w30857
- S. A. Alowais et al., "Revolutionizing healthcare: the role of artificial intelligence in clinical practice," BMC Medical Education, vol. 23, no. 1, Sep. 2023, doi: 10.1186/s12909-023-04698-z. Available: https://doi.org/10.1186/s12909-023-04698-z
- "General Assembly adopts landmark resolution on artificial intelligence," UN News, Mar. 21, 2024. Available: https://news.un.org/en/story/2024/03/1147831
- "Healthcare Data Breach Statistics," Jul. 18, 2024. Available: https://www.hipaajournal.com/healthcare-data-breach-statistics/. [Accessed: Aug. 05, 2024]
Disclaimer: Our content serves informational purposes only and shouldn't replace the advice and services of qualified professionals. While we strive for accuracy, we cannot guarantee an entirely error-free experience. C8 Health will not be liable for any losses or damages resulting from the use of our content.